Building a full-stack robotics company in the age of large AI models
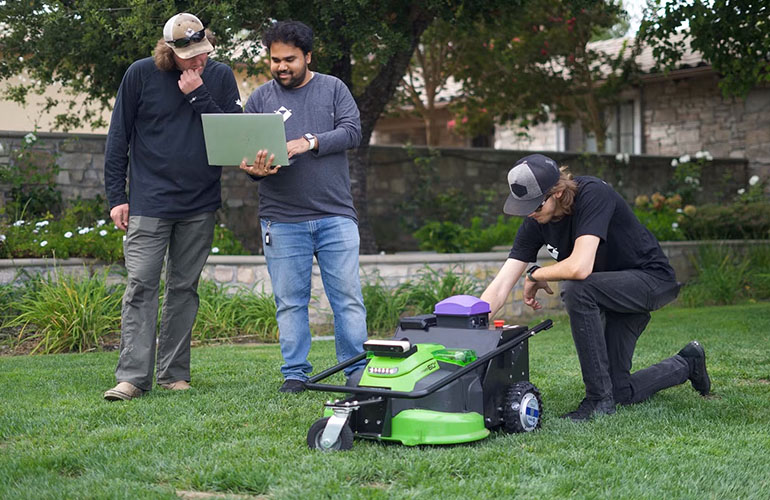
Electric Sheep is a provider of autonomous large-scale outdoor maintenance. | Source: Electric Sheep
In today’s fundraising environment with its intense focus on cash flow positive business models, robotics entrepreneurs are left navigating some thorny challenges: high CapEx costs, workflow and change management, and long enterprise deployment cycles to name a few.
This is further exacerbated by the choice of a business model such as RaaS (Robotics as a Service), which on paper looks promising, but ends up forcing software-like expectations on what is fundamentally a very different business opportunity.
However, in industries such as maintenance services that heavily depend on labor and are already mechanized, an alternative approach exists – if we can reframe the opportunity and promise of automation.
Rethinking GTM: get paid to deliver outcomes, and use your profits to build robots to improve your own margins
By acquiring established service-based companies or entering into service contracts directly with customers, robotics entrepreneurs can fund their operations directly from customer revenue. Case in point – Amazon has built 750,000 robots for itself and can scale them profitably. On the other hand – most RaaS companies bleed cash into eternity, even after going public. Shopify bought Six River Systems for half a billion dollars and sold it to Ocado for 12 million dollars.
Who would you rather be: the robotics startup that hopes some incumbent will select you after pilot purgatory, or a behemoth that rolls up and dominates an entire industry?
The importance of this strategy has been further strengthened by the rise of Large ML Models (such as LLMs and VLMs behind ChatGPT and Palm-E). Just as LLMs have started to transform knowledge work – we are now beginning to see the emergence of Vision and Sensor Data-driven Large Models that will transform manual work as we know it.
The power of Large Models is derived from the trifecta of Data, Compute, and Reinforcement Learning from Human Feedback (RLHF). Though computing power is an invaluable asset, it will largely become a commodity. It stands to reason that Data from real-world operations will be the key driver to develop universal embodied intelligence which will eventually drive all robots.
Alongside the Data, building an ongoing training and fine-tuning environment to shape these models will be key. (If you want proof that Data Quality or RLHF is the key differentiator – compare GPT4 vs any open source model – OpenAI’s secret weapon is their army of Scale.AI and other RLHF resources.)
With the shift from classical robotics to large models, it’s clear that data acquisition and continual fine-tuning are the bedrock on which future robotics will be built.
Register now so that you don't miss this exciting event.
Blueprint for Vertically Integrated Robotics: A Deeper Dive
At Electric Sheep Robotics we are actively applying this thinking towards automating the $250B outdoor maintenance services industry (think mowing lawns, sweeping parking lots, repairing sidewalks etc – all the boring stuff that keeps the forces of entropy from slowly destroying civilization). Our rapid growth over the past couple of years has provided us with valuable insights, which we would like to share with you here:
We have leveraged our equity to raise acquisition capital
As opposed to equity, which can be expensive, debt is much better suited to buy companies that are cash flow positive. This has allowed us to dramatically improve the efficiency and profitability of our revenue growth. The journey to find compatible companies is fairly straightforward for our industry – private equity has been actively consolidating the outdoor services industry for the past 20 years. KKR in fact created BrightView (NYSE: BV) – which is a $3B ARR behemoth. Brightview was formed over time by rolling up several smaller companies ranging from $5MM all the way to $50MM ARR.
We have focused on some core geographies such as the South of the US – which have plenty of maintenance revenue (In our industry – maintenance tasks are largely mechanized using equipment. As our ML models mature – this translates to adding a layer of automation to an increasing number of tasks.)
When integrating a business we have considered other cultural issues as well such as the tech-savviness of the existing team, the commitment of the seller towards enabling automation, and the ability to stay on to help with the transition.
We have committed to building a full-stack, multidisciplinary team:
We have focused on people with a growth mindset and over-indexed on those who were willing to cut across domains and were willing to roll up their sleeves to do whatever it took to ship. This often meant promoting junior employees with a vision to build robotics of the future vs hiring senior leadership from larger companies.
To run acquired businesses, we have sought operations managers who implemented IT systems or other IOT technology to optimize labor management and fleet operations. We have found them to have battle scars, and as a result, a more nuanced understanding of the people, process and product problems that have to be overcome when deploying automation.
For example, we decided very early on to remove all attempts to introduce a phone app or any digital UX onto our robots. As we scale our deployments we continually obsess over small ways to improve the UX – how to withstand the abuse the robot would take as a piece of landscaping equipment, how it will be transported, how it will be washed (in some cases, actively sabotaged by the unenthusiastic crew!).
We view robots as a tool to improve margins:
Instead of viewing robots as products for sale, we envision them as strategic tools to enhance operational efficiency and profitability. This forces us to focus on function and usefulness and avoid falling into useless product feature traps. Since we build for ourselves – we know exactly what we need to improve our own efficiency.
What this has translated to in practice is that we can release imperfect robots that may not have the fanciest UX, packaging, or bring-up procedures. Our robots aren’t the most polished looking, but they get the job done and have allowed us to deploy and iterate at scale.
Also, in every site we are deployed at, we have identified an internal ops team member to act as the voice of the customer. Pleasing this ops member is the only goal for the engineering team, and it forces them to repeatedly confront the boring details that make a great product and make tradeoffs that directly contribute to the bottom line, instead of improving or developing things that may not have immediate ROI.
Our ultimate goal is to keep building a Data and Deployment Flywheel:
What makes Tesla such a formidable force, and why is it unlike any other AV automation effort out there? Two words – Data Engine. It’s not the models, compute, or sensors – it’s ultimately the feedback loop that drives the success of ML models. Here are some things we have kept in mind as we build our data engine:
First, we have come to realize data isn’t oil as much as it is a carefully curated art collection — owning a thousand random sketches doesn’t compare to having a few masterpieces that can truly illuminate your understanding. Before we decide what data to use or collect, we apriori run small batch experiments to understand what signals we really need to improve.
Second, we have designed our data engine to be robust to operator error and to happen without any explicit actions needed on the part of the operator. We synchronize our robot charging with our data upload and have also made our engine robust to missing a few days or even weeks of feedback.
Third, We have built out a real-time communication system that allows our field operators to log issues as they happen to notice them. The key is to not burden field operators with onerous logging requirements that detract from their other tasks and impact operating margins. We also combine this with a weekly triage meeting that allows operators and engineers to talk to each other and co-evolve better ways to use the robot is key.
We have set internal expectations for imperfect, progressive automation:
Most robotics efforts try to deploy the twin cost burners of CapEx and sales effort really fast. In our early days, we tried a RaaS GTM, and we had the same issues. We found that CapEx burn was driven by external customer expectations to deliver against service level agreements. And sales burn was driven by investor expectations to deliver against contracted ARR.
When scaling robotics companies it is key to internalize that atoms are statistical beasts while classical algorithms or software are not. The ultimate prize for robotics success is a much bigger and more defensible moat than any software.
In our case, our ultimate prize is an embodied AI brain that can do all manner of outdoor autonomy tasks. We take the long view on this, and equally importantly we have found it helpful to build a shared point of view with our investors and customers and employees.
We are clear on our ultimate end goal – and the tradeoffs/ benefits to get there:
We have decided to consolidate the whole industry and capture all the value for ourselves.
This works out well for industries where there is recurring revenue and a steady stream of cash that can be used to fund and scale automation. Also, this is a unique way for a robotics company to build up to Tesla and Amazon levels of scale and profitability while building up a massive data and distribution moat. In the coming decades when ML will eat everything, this (to us) feels like a no-brainer.
The tradeoffs to consider here are: how do you match the right sources of capital to the right activity? Traditionally maintenance services businesses command lower multiples but are also inherently profitable and steady, and acquisition is best serviced by debt.
Equity should be viewed as a means to de-risk the engineering and fund the more moonshot research around models of the future. Getting a combination of equity and debt is a tradeoff that we consider as we continue to scale, and this informs how we invest in RnD, GTM, how we value cash flow and acquired growth over organic, etc.
In conclusion
There are many different ways to build a robotics company. Our approach may or may not make sense for your particular industry, but it’s worth considering some broad takeaways.
If your thesis, like ours, is that robotics is going to be a data-intensive industry that will be dominated by large ML models and reinforcement learning, then it stands to reason that your goal is to acquire data and distribute in the most capital-efficient way possible. The hardware and the software will figure itself out in the process of gradual evolution.
Thinking of yourself as a service provider, data aggregator, and builder of your own foundation model for your robotics application, as opposed to a robot maker, is one way to get here.
About the Author
Nag Murty is the founder and CEO of Electric Sheep Robotics and a 2x deep-tech entrepreneur.
The post Building a full-stack robotics company in the age of large AI models appeared first on The Robot Report.
from The Robot Report - Robotics News, Analysis & Research https://ift.tt/Q1WUV8s
via artificialconference
Comments
Post a Comment